Slides for the keynote speaker are available here.
Venue
Academic Hall (Room 702)
Central University of Finance and Economics
39 South College Road, Haidian District, 100081 Beijing, China
Date
Saturday, November 18, 2017
Invited Speakers
- Rob Hyndman, Monash University
- Junni Zhang, Peking University
- Lei Song, JD.com
- Hui Bu, Beihang University
- Yanfei Kang, Beihang University
- Feng Li, Central University of Finance and Economics
Registration
No registration fee is charged for all participants, but participants should register for the workshop via this link (or scan the following QR code) before November 15, 2017.
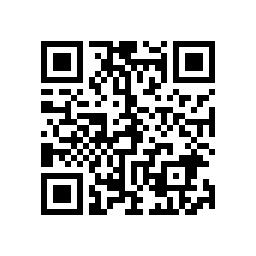
Contact Us
If you have any questions, please contact the local organizer Feng Li <Email: feng.li@cufe.edu.cn>
Program
09:00-12:00 Keynote Lecture on Forecasting
Speaker: Rob Hyndman, Monash University
- Topic I: Forecast accuracy and evaluation
How do we decide which forecasting method is the best for our time series? There have been several measures of forecast accuracy proposed, which will be discussed. We will also look at how these can be used to select a forecasting model for a given time series.
- Topic II: Automatic time series forecasting: ETS, ARIMA, STLM, TBATS, FASSTER
Many applications require a large number of time series to be forecast completely automatically. For example, manufacturing companies often require weekly forecasts of demand for thousands of products at dozens of locations in order to plan distribution and maintain suitable inventory stocks. In these circumstances, it is not feasible for time series models to be developed for each series by an experienced analyst. Instead, an automatic forecasting algorithm is required. I will describe some of the best automatic forecasting algorithms that are widely used in practice, as well as a new method that has only been recently proposed. All of the algorithms are implemented in R.
- Topic III: Hierarchical forecasting
Time series can often be naturally disaggregated in a hierarchical or grouped structure. For example, a manufacturing company can disaggregate total demand for their products by country of sale, retail outlet, product type, package size, and so on. As a result, there can be millions of individual time series to forecast at the most disaggregated level, plus additional series to forecast at higher levels of aggregation. A common constraint is that the disaggregated forecasts need to add up to the forecasts of the aggregated data. This is known as forecast “coherence”. When we turn incoherent forecasts into coherent forecasts, we call it “forecast reconciliation”. I will show that the optimal reconciliation method involves fitting a linear regression model where the design matrix has one column for each of the series at the most disaggregated level. But with huge numbers of time series, the model is impossible to estimate using standard regression algorithms. I will show how the hts package for R solves this problem.
13:30-15:30 Demographic and Economic Forecasting, Chaired by Feng Li
- 13:30-14:10 Bayesian demographic estimation and forecasting
Speaker: Junni Zhang, Peking University
Abstract: There is a natural fit between Bayesian statistics and demographic estimation and forecasting. Bayesian methods can cope with complex models and noisy data, which are common in demographic estimation and forecasting. Bayesian methods also provide rich measures of uncertainty. In the talk, we will describe a long-term project to develop methods and software for Bayesian demography. The project emphasises disaggregated estimates and forecasts. We will give an overview of our framework, and present some illustrative results.
- 14:10-14:50 JD Smart Supply Chain Driven by AI
Speaker: Lei Song, Senior Machine Learning Engineer, JD.com
Abstract: In JD, AI technologies have been applied in many areas of our bussiness, for instance SKU recommendation, drones, automatic warehouses etc. In this talk, I will focus on another important application of AI in JD, namely, supply chain management. I will show you how we leverage AI technologies to drive every process of our smart supply chain, which includes demand forecast, automatic replenishment, SKU allocation, markdown etc.
- 14:50-15:30 Forecasting time series with complex seasonal patterns using singular spectrum analysis
Speaker: Hui Bu, Beihang University
Abstract: Time series from business and economics may have multiple seasonal components, which may be difficult to identify when multiple seasonal autoregressive integrated moving average (ARIMA) models are built. To solve this problem, we propose a specification method for multiple SARIMA model, called SSA-SARIMA procedure, that draws on the advantages of singular spectrum analysis (SSA) and the Box-Jenkins standard procedure of the seasonal ARIMA (SARIMA) model to reduce the human influence. This paper utilizes monthly passenger throughput data of the Hong Kong and San Francisco airports to test the proposed SSA-SARIMA modeling method. We compare the out-of-sample forecasting performance of multiple SARIMA model following SSA-SARIMA procedure and the standard Box-Jenkins procedure, SSA recurrent forecasting method, hybrid model of SSA and SARIMA and hybrid model of wavelet and SARIMA. The empirical results show that double seasonal ARIMA models identified by our method better fit the data and improve their out-of-sample forecasting performance.
15:50-17:30 Probabilistic Forecasting, Chaired by Yanfei Kang
- 15: 50-16:30 Probabilistic hierarchical forecasting
Speaker: Rob Hyndman, Monash University
Abstract: Forecasting hierarchical time series has been of great interest in recent years. However, the literature has mainly focused on obtaining point forecasts that are coherent across a hierarchy. Instead, I will focus on the problem of producing probabilistic forecasts for hierarchical time series. First, we will look at how to define coherency in a probabilistic setting. Then we will look at some results for probabilistic coherence assuming Gaussian forecast densities. Finally, we will relax the Gaussian assumption and propose a novel, non-parametric approach to generate coherent forecasts. This entails simulating future sample paths of the whole hierarchy by incorporating bootstrapped in-sample errors and reconciling these so that they become coherent. We evaluate both approaches via extensive Monte Carlo simulations.
- 16:30-17:00 Improving forecasting performance using covariate-dependent copula models
Speaker: Feng Li, Central University of Finance and Economics
Abstract: Copulas provide an attractive approach for constructing multivariate distributions with flexible marginal distributions and different forms of dependences. Of particular importance in many areas is the possibility of explicitly forecasting the tail-dependences. Most of the available approaches are only able to estimate tail-dependences and correlations via nuisance parameters, but can neither be used for interpretation, nor for forecasting. Aiming to improve copula forecasting performance, we propose a general Bayesian approach for modeling and forecasting tail-dependences and correlations as explicit functions of covariates. The proposed covariate-dependent copula model also allows for Bayesian variable selection among covariates from the marginal models as well as the copula density. The copulas we study include Joe-Clayton copula, Clayton copula, Gumbel copula and Student’s t-copula. Posterior inference is carried out using an efficient MCMC simulation method. Our approach is applied to both simulated data and the S&P 100 and S&P 600 stock indices. The forecasting performance of the proposed approach is compared with other modeling strategies based on log predictive scores. Value-at-Risk evaluation is also preformed for model comparisons.
- 17:00-17:30 Forecasting using time series feature spaces
Speaker: Yanfei Kang, Beihang University
Abstract: This paper investigates the diversity of the M3 dataset used by most forecasting researchers and shows how a time series dataset can be visualized, with each time series being a point in a two-dimensional space. According to the No-Free-Lunch theorem, there is no one time series forecasting method that will always perform best. Given that the statistical features of time series have an impact on their forecasting accuracies, we aim to examine how those features influence forecasting method performances through simulations from mixture autoregressive (MAR) models, which enables us to predict the performances of the forecasting methods on the M3 data and select the best forecasting method. Finally, we propose a new method for generating new time series with controllable characteristics with the aim of enriching the diversity of the feature space, which provide potentially more insights than the current space.